What Role Does Artificial Intelligence Play in Achieving Sustainability?
Technology can help us make giant leaps. Check out how Artificial Intelligence and Machine Learning can help us become more Sustainable.
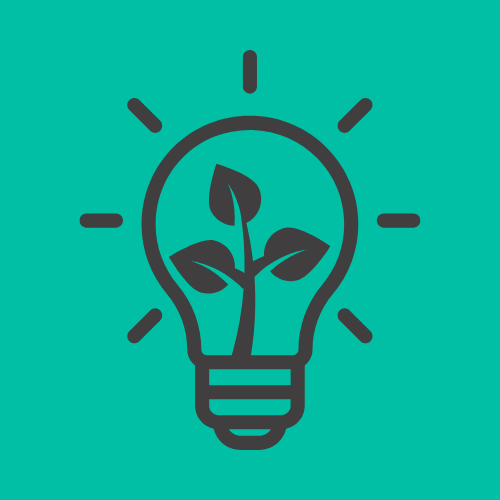
Machine learning is a subset of artificial intelligence (AI) which, at the time of writing, has little to do with climate change or our efforts to combat excessive waste. However, many predict that is about to change. Recent developments in machine learning have genuine social value: doctors now use machine learning to make more accurate diagnoses, and self-driving cars are becoming increasingly widespread. Machine learning continues to transform industries and, if we utilize it correctly, it will become a vital tool in our efforts to lead better, more sustainable lives.
The issue, however, is that many of us don’t know what machine learning is and can’t really imagine what a future led by AI might look like. We know what it means to put recycling in the correct bins, and we just have to turn on the news to see the direct impact of excessive waste. But what is machine learning? And how can it help us reduce waste and emissions?
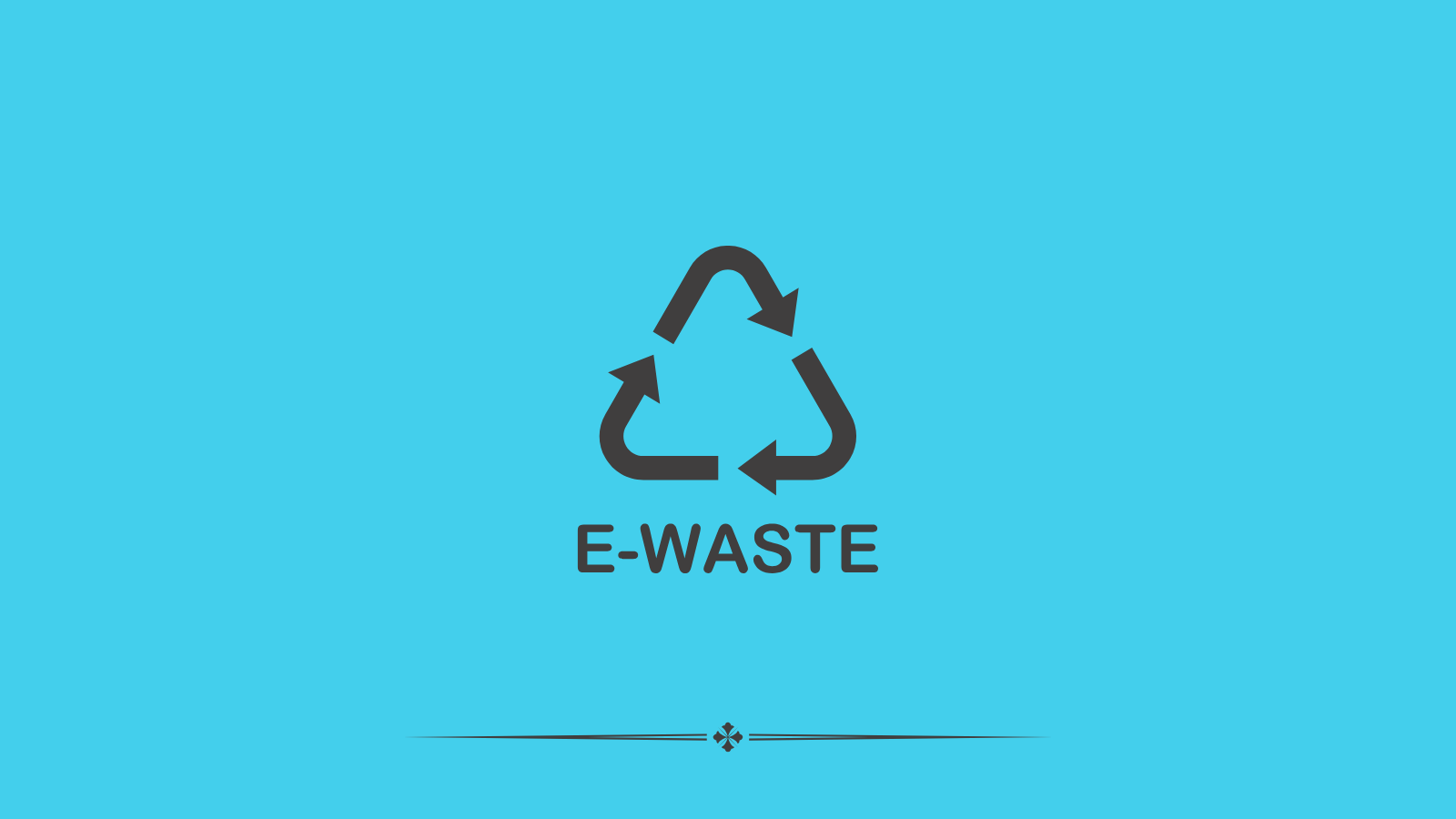
E-Waste and its Effect on Our World
Electronic waste is a disturbing by-product of our rapidly evolving, tech-driven worlds. When an electronic product breaks or is deemed to have outlived its usefulness, it becomes e-waste. Every phone, laptop, household product, or electronic product that we throw away adds to the already staggering pile of e-waste that is held in landfills.
A recent UN study estimated that, in 2019 alone, we threw away 50 million tonnes of e-waste, but only recycled around 10 million metric tonnes. The issue of e-waste is clearly spiraling out of our control and presents a significant threat to the environment and vulnerable human populations worldwide. Simply, we need intelligent solutions that are capable of dealing with the growing issue of e-waste. Many believe those solutions will come from artificial intelligence technologies which are capable of computing and analyzing the massive amounts of data involved in e-waste.
Defining AI + Machine Learning
For those of us less familiar with artificial intelligence and machine learning, it might be helpful to take a step back and provide a few definitions.
Artificial Intelligence
Nils J. Nilsson defines AI as “that activity devoted to making machines intelligent, and intelligence is that quality that enables an entity to function appropriately and with foresight in its environment.”
In other words, AI is the use of computing systems to generate intelligence that imitates—and sometimes exceeds—human thought. More specifically, AI’s usefulness lies in the fact that computers are capable of storing and analyzing massive amounts of data more efficiently than humans can. As such, AI is better suited to completing tasks that involve large amounts of data—a human can’t sift through 50 million tonnes of e-waste a year, but a correctly programmed AI certainly can.
Machine Learning
Machine learning is a subfield of AI which mimics human learning. Just as we learn things through trial and error, machine learning requires the input of data to learn. This data is collected through data labeling. The neat thing about machine learning is that it does not require a human at every step: when done correctly, machine learning allows computers to learn and adapt on their own. This is useful as it means computers—which can quickly process vast amounts of data more efficiently than humans—can learn and respond to varied inputs to create solutions that draw from massive volumes of data.
Computer scientist Hilary Mason emphasizes that machine learning’s real value is that “it gives us the ability to learn things about the world from large amounts of data that we as human beings can’t possibly study or appreciate.” Mason also explains that the challenge of machine learning isn’t in simply creating programs but rather in finding ways to effectively apply machine learning to the real world and all its chaotic, unpredictable problems.
As far as unpredictable, chaotic problems go, climate change is something of a final boss. As such, it requires software engineers who specialize in the subfield of machine learning. Machine learning engineers vary slightly from software engineers, as they are required to take on multidisciplinary approaches to data science. Simply, machine learning engineers have to consider the real world that exists beyond the program and must find ways to adapt to meet the demands of society.
Machine Learning’s Vital Role in Reducing E-Waste
Machine learning is already being applied to sustainability projects. Tech giants like Google and IBM are using AI to reduce the overall impact of their operations on the environment, and are now leveraging AI models to advocate for sustainable development.
In the effort to reduce our e-waste and create more sustainable futures, it is clear that we need intelligent AI which is capable of accurately identifying and sorting the 50 million tonnes of e-waste we throw away yearly. As such, there is increasing interest in creating machine-learning programs to tackle e-waste. These developments—in unison with other exciting technologies like augmented reality — will increase the overall efficacy of our efforts to recycle a greater proportion of e-waste.
AI and machine learning can be leveraged on both large and small-scale recycling projects. Companies are already developing waste management AI capable of sorting waste at twice the speed of humans. On a smaller scale, waste bins that contain AI recognition technologies are currently being prototyped internationally, and in Bournemouth, UK, the “Leave Only Footprints” campaign is using drones to gather essential data for waste management AI.
Looking Ahead
For the time being, reducing our waste is a vital component in fighting climate change and reducing environmental damage. But, with new waste-reduction technologies being trialed and brought into widespread usage, we can feel some hope that the effort to reduce waste and reverse climate change will progress beyond individual responsibility and inefficient recycling bins. Machine learning, in tandem with other high-tech developments, offers us a way to take on the unimaginable scale of e-waste. In the comings years, machine learning will create the deep neural networks of AI knowledge necessary for more efficient recycling schemes—in a strange way, that thought is deeply comforting.
The Future of Artificial Intelligence in Achieving Sustainability
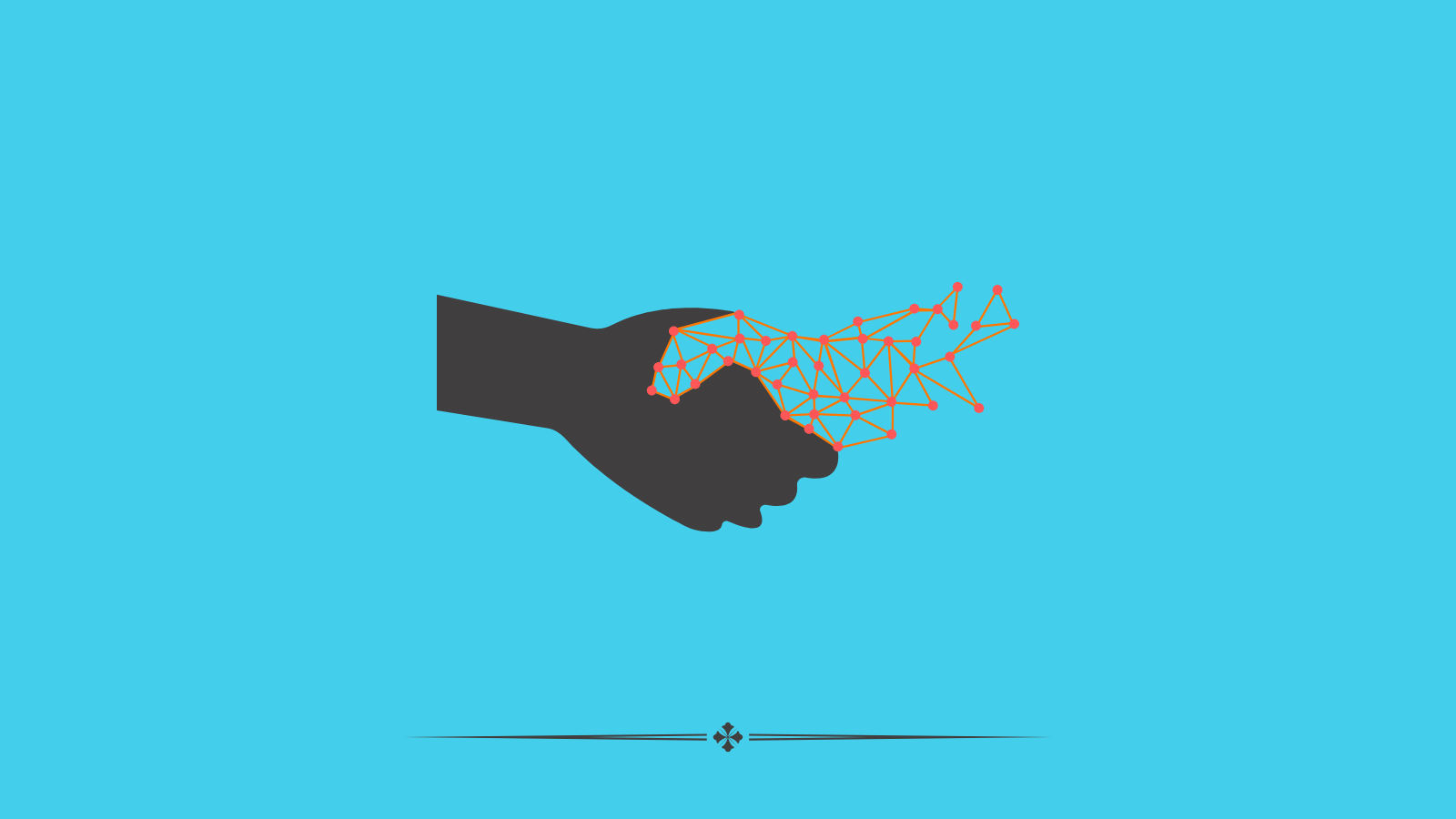
Artificial intelligence, with its ability to process vast amounts of data and deliver actionable insights, is revolutionizing sustainability efforts across multiple industries. Here’s a closer look at how AI contributes to key areas of environmental stewardship:
1. Energy Optimization
AI algorithms are transforming the energy sector by improving efficiency and reducing waste.
- Smart Grids: AI-powered grids can predict energy demand, balance loads, and integrate renewable energy sources more effectively.
- Energy Efficiency: Machine learning tools analyze consumption patterns in real time, helping businesses and households reduce unnecessary energy usage.
- Renewable Energy Forecasting: AI models accurately predict solar and wind energy output, making renewable energy more reliable and scalable.
2. Reducing Carbon Footprints
- Carbon Emission Tracking: AI tools allow companies to monitor and measure carbon emissions with precision, helping them identify areas for improvement.
- Sustainable Logistics: AI optimizes supply chain routes and vehicle fleets to minimize fuel consumption and emissions.
- Green Infrastructure Design: Urban planners use AI simulations to create energy-efficient buildings and sustainable cities.
3. Waste Management
AI is revolutionizing how we manage waste by automating sorting processes and promoting recycling.
- Smart Recycling Systems: AI-powered robots can identify and sort recyclable materials faster and more accurately than humans.
- Predictive Analytics for Waste Reduction: Businesses use AI to forecast waste generation and develop strategies to minimize it.
AI and Climate Change: A Game-Changer
Climate change is one of the most pressing issues of our time, and AI is proving to be a critical tool in combating its effects.
- Climate Modeling: Advanced AI models analyze weather patterns, predict climate changes, and help policymakers prepare for extreme weather events.
- Forest Monitoring: AI-powered drones and satellites detect illegal deforestation and monitor reforestation efforts in real time.
- Carbon Capture Technology: AI enhances the efficiency of carbon capture and storage systems by optimizing operations and reducing costs.
Agriculture and Food Sustainability with AI
The agriculture sector faces mounting pressure to produce more food while conserving resources. Here’s how AI is making farming smarter and more sustainable:
- Precision Farming: AI sensors monitor soil health, water levels, and crop conditions, allowing farmers to apply resources only where needed.
- Reducing Food Waste: AI tools predict harvest yields and optimize food distribution, reducing waste across the supply chain.
- Alternative Proteins: AI accelerates the development of plant-based and lab-grown meats, reducing the environmental impact of traditional livestock farming.
Challenges and Ethical Considerations
While the potential of AI in achieving sustainability is immense, there are challenges to consider:
- Energy Consumption of AI: The computational power required for AI systems can consume significant energy, creating its own carbon footprint.
- Data Privacy: Sustainability solutions often involve tracking individual or organizational behaviors, raising concerns about privacy and data security.
- Equitable Access: Ensuring that AI-driven sustainability tools are accessible to all regions and income levels is crucial to avoid exacerbating inequalities.
The Future of AI in Sustainability
The journey toward sustainability is far from over, but AI is poised to play a central role in driving progress. Here are some emerging trends to watch:
- Circular Economy Models: AI will support the transition to a circular economy by optimizing resource recovery and reuse.
- Sustainable AI Development: The rise of energy-efficient AI models will minimize the environmental impact of technology itself.
- Global Collaboration: AI platforms will facilitate international cooperation by providing shared data insights and solutions to global sustainability challenges.
Conclusion: A Green Future with AI
The integration of AI into sustainability efforts represents a promising step toward addressing the environmental challenges of our time. From optimizing energy usage to combating climate change and revolutionizing agriculture, AI offers tools that are as innovative as they are impactful. However, achieving true sustainability will require a balance of technological advancements, ethical practices, and global collaboration.
As AI continues to evolve, its role in shaping a greener, more sustainable future will only grow. The question is no longer whether AI can help us achieve sustainability but how quickly and effectively we can leverage its potential to create lasting change.
Take Action Today
Organizations and individuals alike must embrace AI-driven sustainability solutions to contribute to a healthier planet. The future is here, and it’s powered by AI—let’s make it green.
This article was written by Jori Hamilton
Create more and better content
Check out the following resources and Grow!