Exploring the Impact of Machine Learning on Financial Inclusion
The integration of machine learning (ML) into financial services is transforming how institutions manage credit scoring. This transformation is especially crucial for promoting financial inclusion, which is vital for economic growth.
By leveraging ML, banks and other financial bodies can provide credit opportunities to underserved communities, broadening economic participation and stability.
Chapters
The Evolution of Machine Learning in Credit Scoring
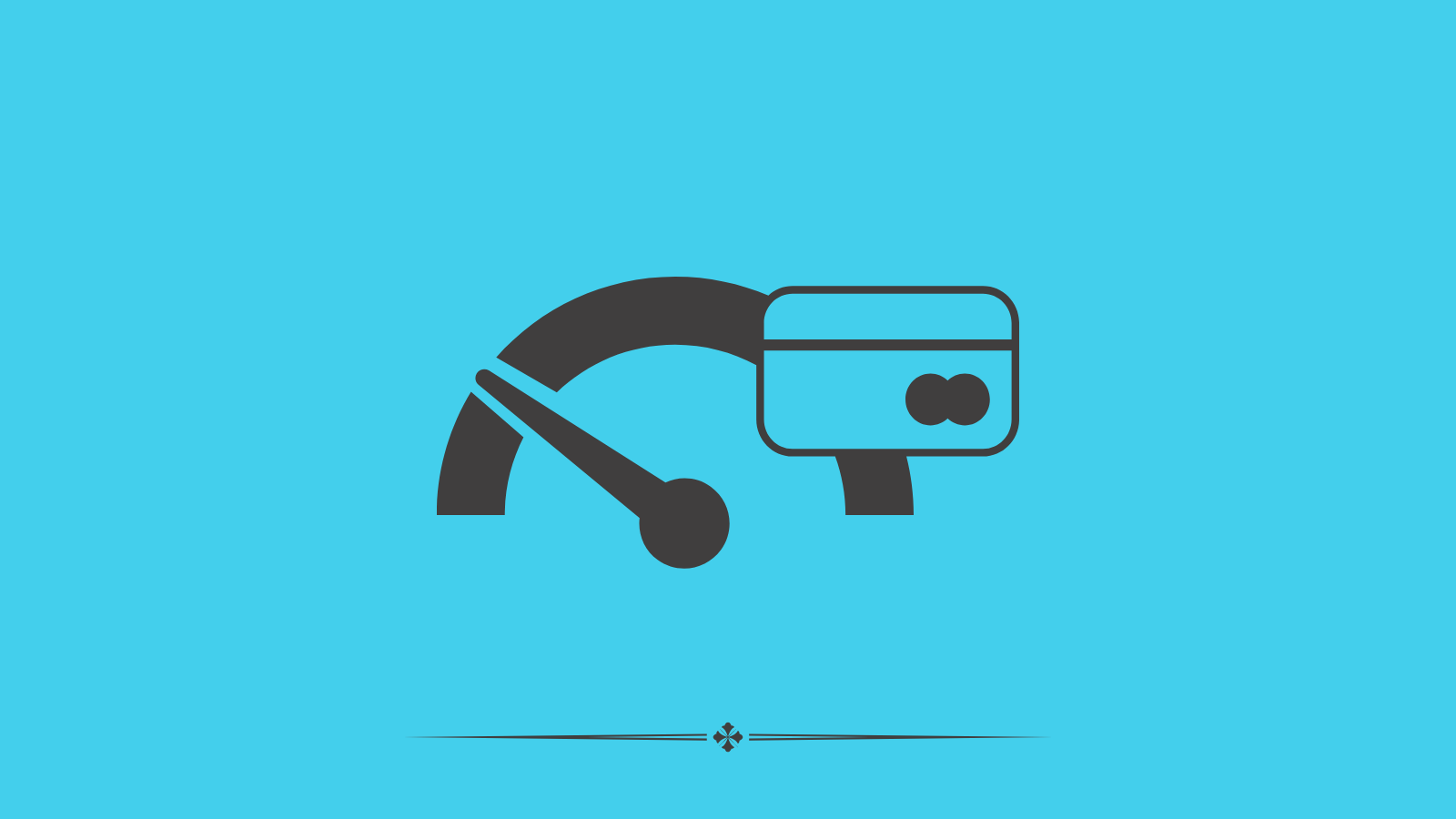
Machine learning is reshaping credit scoring by utilizing a range of both conventional and unconventional data sources. Traditional systems often miss potential borrowers who may be creditworthy but lack extensive financial histories. ML algorithms excel in extracting insights from diverse data sets, including transaction histories, social media activity, and even geographic information. This ability not only enhances decision-making processes but also helps in identifying new customer segments that were previously invisible under traditional systems.
Benefits of ML for Financial Inclusion
- Broader Data Analysis: ML can process complex and varied data sets at an unprecedented scale, from rental histories to remittance data, providing a more comprehensive view of a borrower’s financial behavior.
- Access for the “Invisible” Borrowers: Many individuals do not have a formal credit history but do have reliable income streams. ML models can identify and predict the risk associated with these users, facilitating broader access to financial products.
- Objective Risk Assessment: ML reduces human errors and biases by consistently applying the same criteria across all applications, which supports a more equitable lending environment.
Enhance your understanding of machine learning credit scoring with Svitla’s comprehensive guide. Benefit from their in-depth expertise in AI, Machine Learning, Computer Vision, and Natural Language Processing to leverage cutting-edge technology in your credit scoring processes. Learn more about how these advanced techniques can be applied effectively in the financial sector.
Challenges and Ethics
The adoption of ML in credit rating is difficult. The discussion centers on data privacy and algorithmic prejudice. ML models must be open and intelligible to sustain customer confidence. To safeguard customers and the financial system, regulatory compliance must be properly handled.
Expanding Impact with Real-World Applications
Globally, innovative financial companies are leveraging ML to transform credit accessibility. In India, startups are using ML to provide small loans to people based on digital payment histories. In Latin America, fintech firms use psychometric data as part of their credit evaluation process to serve customers who lack traditional credit histories.
What is Machine Learning
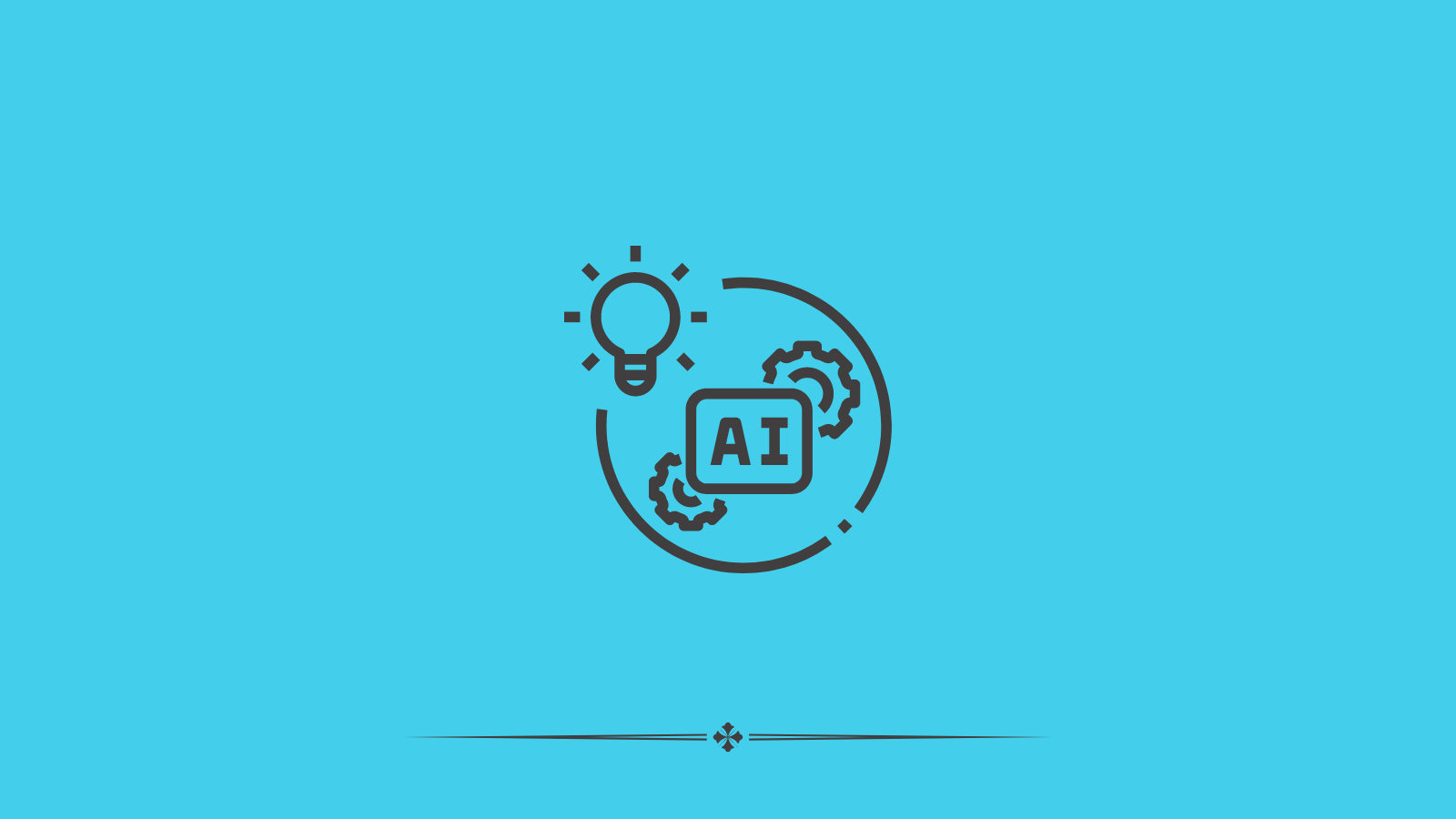
Machine learning is a subset of artificial intelligence (AI) that focuses on the development of algorithms and statistical models that enable computers to learn and make decisions from data without being explicitly programmed. Here are the key components and concepts of machine learning:
Key Components and Concepts
- Algorithms:
- Algorithms are the mathematical procedures or formulas used to perform the learning process.
- Examples include decision trees, neural networks, support vector machines, and k-nearest neighbors.
- Data:
- Data is the core component on which machine learning models are trained. It can be structured (like databases) or unstructured (like text or images).
- Models:
- Models are the output of the learning algorithms after being trained on data. They are used to make predictions or decisions.
- Training:
- Training is the process of feeding data into an algorithm to create a model. The algorithm adjusts its parameters to minimize errors in predictions.
- Features:
- Features are individual measurable properties or characteristics of the data used in the learning process.
- Labels:
- Labels are the outcomes or target variables in supervised learning. They are used to guide the learning process.
Types of Machine Learning
- Supervised Learning:
- Involves learning a function that maps input data to the desired output using labeled data.
- Examples: Classification (e.g., spam detection), Regression (e.g., predicting house prices).
- Unsupervised Learning:
- Involves finding hidden patterns or intrinsic structures in input data without labeled responses.
- Examples: Clustering (e.g., customer segmentation), Association (e.g., market basket analysis).
- Semi-Supervised Learning:
- Combines a small amount of labeled data with a large amount of unlabeled data during training.
- Reinforcement Learning:
- Involves learning how to act by performing certain actions and receiving rewards or penalties.
- Examples: Game playing (e.g., AlphaGo), Robotics.
Applications of Machine Learning
- Healthcare:
- Disease prediction, medical image analysis, personalized treatment plans.
- Finance:
- Fraud detection, algorithmic trading, credit scoring.
- Marketing:
- Customer segmentation, personalized recommendations, sentiment analysis.
- Transportation:
- Self-driving cars, traffic prediction, route optimization.
- Natural Language Processing (NLP):
- Language translation, speech recognition, text generation.
Challenges and Considerations
- Data Quality and Quantity:
- The performance of machine learning models heavily depends on the quality and quantity of the data used for training.
- Overfitting and Underfitting:
- Overfitting occurs when a model learns the training data too well, including noise and outliers, leading to poor performance on new data.
- Underfitting occurs when a model is too simple to capture the underlying patterns in the data.
- Model Interpretability:
- Some models, like neural networks, are considered “black boxes” and can be difficult to interpret.
- Bias and Fairness:
- Ensuring that models do not perpetuate or amplify biases present in the training data.
Machine learning is a rapidly evolving field with a wide range of applications and significant impact across various industries. Its success relies on the careful design of algorithms, the availability of quality data, and addressing ethical considerations.
FAQ Section
Q: How does machine learning improve credit scoring?
A: ML improves credit scoring by analyzing a broader array of data points more accurately and quickly than traditional models, thus identifying creditworthiness more effectively.
Q: What are ML credit scoring risks?
A: Data breaches, biases perpetuated by poorly curated data, and opaque automated conclusions are major hazards.
Q: Can machine learning minimize credit scoring biases?
A: ML models may discover and minimize unconscious biases using data and patterns rather than human judgment if correctly developed.
Machine learning is making credit scoring more accessible, fair, and efficient, boosting financial inclusion. As these technologies advance, governments, technologists, and financial institutions must work together to utilize them ethically and responsibly to maximize advantages and minimize risks.
Create more and better content
Check out the following resources and Grow!